Machine learning may help in earlier ankylosing spondylitis diagnosis
Goal is to identify patterns that mark people who develop the disease
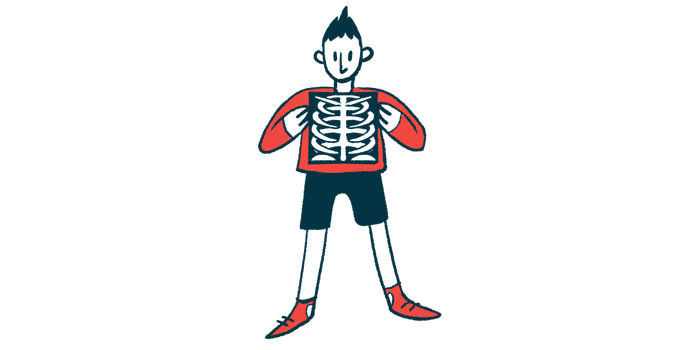
Machine learning methods may help in the early detection of ankylosing spondylitis (AS), a new study reported.
“It’s fantastic to see the cutting-edge role that machine learning can play in the early identification of patients with health conditions such as AS … Though it is in its early stages, machine learning clearly has the potential to transform the way that researchers and clinicians approach the diagnostic journey, bringing about benefits to patients,” Kieran Walshe, director of Health and Care Research Wales, said in a press release.
The study, “Predicting a diagnosis of ankylosing spondylitis using primary care health records–A machine learning approach,” was published in the journal PLOS One.
Potential seen, but more work needed to predict those at disease risk
Machine learning can help with early disease detection and diagnosis that are essential for good care, allowing doctors to “refer patients more effectively and efficiently,” said Jonathan Kennedy, the study’s lead author at the National Centre for Population Health & Wellbeing Research in Wales.
“However, machine learning is in the early stages of implementation. To develop this, we need more detailed data to improve prediction and clinical utility,” Kennedy said.
Since AS symptoms like lower back pain are common to various conditions, it is often difficult to identify people at greatest risk of AS. Diagnosing the disease can be a complicated process, generally involving a combination of imaging tests, laboratory tests, and other clinical evaluations. Nonetheless, an early and accurate diagnosis is critical for facilitating proper disease treatment.
“On average, it takes eight years for patients with AS from having symptoms to receiving a diagnosis and getting treatment. Machine learning may provide a useful tool to reduce this delay,” said Ernest Choy, a study co-author at Cardiff University.
A team of scientists in the U.K. used machine learning analyses of healthcare records to identify patterns that could help with spotting AS earlier.
“Novel machine learning methods can potentially identify patterns and clusters of terms/data, such as diagnosis, procedures, and medications, which are observed more frequently in people who have a subsequent diagnosis of AS, compared to people who do not receive a diagnosis of AS,” the researchers wrote.
Utilizing information from a national healthcare database in Wales, the scientists identified 543 males and 250 females diagnosed with AS between the ages of 15 and 35. Some of these data were used to construct machine learning models, while the rest was used to test the models. Since AS can vary substantially according to sex, male and female patients were analyzed separately.
Results broadly indicated that factors predictive of AS included a history of pain and treatment with pain medications, X-rays of the spine, or uveitis (inflammation in the eyes). Results were generally consistent across sexes, though women tended to be older at symptom presentation compared to men with back pain on several pain relief medications.
A history of diagnoses with other muscle and/or skeleton diseases, especially at younger ages, was also predictive of a future AS risk. The researchers noted that “women with AS had a greater number of additional musculoskeletal diagnoses and increased numbers of diagnoses from hospitals,” suggesting “that women have a more complicated path to being diagnosed with AS, with multiple tests, diagnoses, and referrals, compared to men with AS.”
Once the machine learning models were constructed, the researchers used statistical tests to assess how accurately they could identify AS. Results generally showed a good accuracy in the initial analysis, but the model was notably less accurate when applied to data from the general population.
Based on the known general public prevalence of AS, the researchers estimated that the ideal positive predictive value for AS in the general population would be 1.44% for males and 0.51% for females. The positive predictive value for the model used was 0.33% for men and 0.25% for women. Of note, positive predictive value is the likelihood that someone with an abnormal test actually has the condition under testing.
“These features identify the female model as being closer to the ‘perfect’ model than the male model,” the researchers wrote. “This implies that the female model is more accurate than the male model at identifying patients with AS.”
These machine learning models likely could be improved by using more detailed patient data, the researchers noted.
“This study indicates that machine learning has the potential to help identify people with AS and better understand their diagnostic journeys through the health system, but we need more detailed data to improve prediction and clinical utility,” they concluded.