Study’s Machine-learning Algorithm Could Help Diagnose AS Sooner
Method relies on blood tests of 9 or 7 factors, including albumin and creatinine
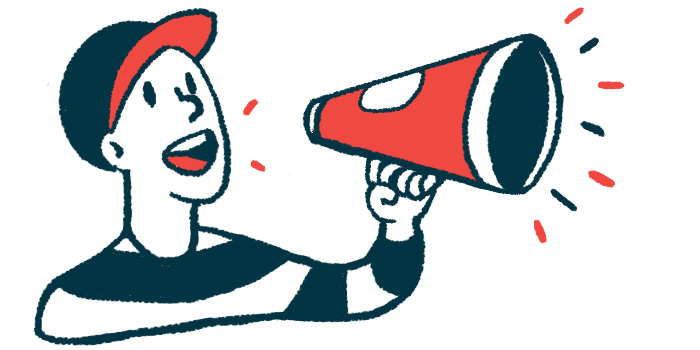
A new algorithm based on artificial intelligence (AI) could help clinicians reach an earlier diagnosis of akylosing spondylitis (AS), a study found.
Using only demographic and clinical data derived from standard blood tests, the method does not require more costly and less widely available imaging techniques, researchers say.
The algorithm “will help clinicians enhance diagnostic efficiency and allow patients to receive systematic treatment as soon as possible,” the China-based researchers wrote, noting that they will continue working to enhance its predictive abilities.
The study, “Development and Validation of a Machine Learning-Based Nomogram for Prediction of Ankylosing Spondylitis,” was published in Rheumatology and Therapy.
AS is a progressive, inflammatory disease mainly affecting the joints of the spine, leading to symptoms of pain and stiffness. No single test can accurately and specifically diagnose the disease. Clinicians typically rely on a physical exam and a thorough medical history to differentiate AS from other conditions that may cause similar symptoms.
Imaging tests, like X-rays or MRI, can also be used to visualize the affected bones and joints. Here, clinicians look for signs of ankylosis, fusion of parts of the spine, or sacroiliitis, inflammation where the spine connects to the pelvis. Both of these are AS hallmarks.
In early disease stages, however, damage to the spine may not have progressed enough to be visible on imaging tests. Further, not all clinics have these imaging tools.
The researchers developed their algorithm as a tool to help identify AS earlier and more accurately, without a need for that type of equipment. Instead, the algorithm would rely on routine lab measurements obtained from blood samples. It was designed to use machine learning, a type of AI that learns from patient data to predict certain outcomes.
The researchers used clinical data from patients diagnosed with AS at the First Affiliated Hospital of Guangxi Medical University in China between 2012 and 2021, as well as data from patients unaffected by the condition, who served as a control group.
A first group of 258 patients and 247 controls were used to “train” the machine-learning algorithm so it could predict an AS diagnosis from clinical and demographic information.
Key factors in predictions
While a range of demographic and blood test measurements differed between AS patients and the control group, the researchers found that nine in particular seemed to have the strongest predictive power. These factors were sex and blood measures of total protein, albumin, red blood cell count, hemoglobin, mean platelet volume, creatinine, aspartate aminotransferase (AST), and erythrocyte sedimentation rate (ESR).
Specifically, most patients in the AS group were male. ESR, a marker of inflammation, was increased in AS samples, as were red blood cell counts and levels of hemoglobin, the protein red blood cells use to carry oxygen throughout the body. Mean platelet volume, an indicator of the production of the small blood cells involved in blood clotting, was lower in AS.
Total protein and albumin, both of which are used in the clinic to monitor nutritional or hydration status, were also increased. AST, a liver enzyme often used to diagnose liver problems, was decreased in AS patients relative to controls, whereas creatinine, a marker of potential kidney dysfunction, was increased.
These nine factors were incorporated into the prediction algorithm. Results showed that it performed well in its ability to predict an AS diagnosis, reaching an area-under-the-curve (AUC) value of 0.88. AUC values, ranging from 0-1, reveal how well a prediction algorithm can perform, with 1 meaning it predicts it right every time and 0 meaning it is wrong every time.
After the model was developed, it was tested again on a “validation” group of 90 additional AS patients and 113 without the disease. The algorithm performed similarly in this group of patients, reaching an AUC of 0.83.
A second, simplified version of the model that accounted for just seven of the nine factors, excluding hemoglobin and total protein, performed similarly to the nine-factor model.
“We established two prediction models that offer the advantages of good performance, high accuracy, and simplicity of use,” the researchers wrote.
The team noted that while the models could be used to aid clinicians in making a diagnosis, “clinicians always have the final word for interpretation based on their domain expertise.”
“In future studies, we will attempt to cover a wide range of clinical variables so that our diagnostic model can be used more accurately in a wider population,” the team concluded.