Machine learning may help diagnose, manage ankylosing spondylitis
Researchers reviewed over a dozen studies that explored its use in the disease
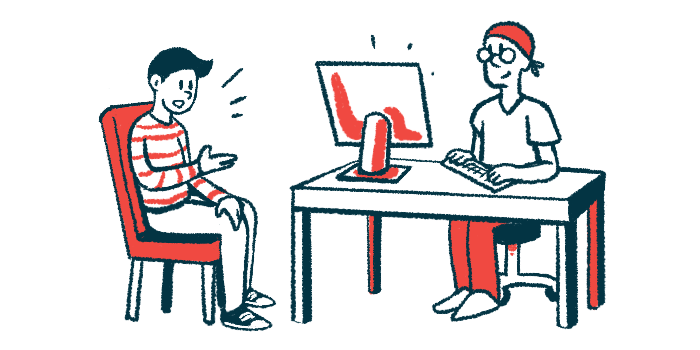
Machine learning and deep learning are already showing promise as tools to help diagnose and manage ankylosing spondylitis (AS), though the field is still in its infancy and more work must be done, a new review paper highlights.
The paper, “Machine Learning and Deep Learning for the Diagnosis and Treatment of Ankylosing Spondylitis- A Scoping Review,” was published in the Journal of Clinical Orthopaedics and Trauma.
Machine learning is a form of artificial intelligence that works by feeding a large dataset into a computer, along with a set of mathematical rules or algorithms that the computer uses to identify patterns in the data. The computer can then apply the patterns it has “learned” to make sense of future datasets. Deep learning is a specific form of machine learning that uses multiple algorithms that act in layers, a setup that mimics how nerve cells fire in the human brain.
AI for AS
Here, a trio of scientists in India reviewed studies published from 2013 to 2023 that explored the use of machine learning or deep learning in AS.
The review highlighted more than a dozen studies that have explored these techniques for various applications in AS. Some have used machine learning to develop tools to help diagnose AS and distinguish it from other inflammatory disorders such as rheumatoid arthritis and osteoarthritis, generally with reasonable accuracy. Other researchers have applied the computer-based analyses to track disease progression and predict how patients will respond to different treatments, namely biologic disease-modifying anti-rheumatic drugs and TNF inhibitors, while other studies have employed deep learning to identify possible disease biomarkers.
The researchers concluded that machine learning/deeep learning “has been used successfully in the identification and screening of AS and differentiating it from the other mimicking conditions.”
“These technologies have also helped in the prediction of progress, and treatment of AS,” they said, but noted that the available studies have limitations. In particular, most used relatively small datasets, often from a single center, to train the machine learning algorithms. Since machine learning works by identifying patterns in datasets, it’s generally most accurate when it has lots of data to “learn” from. The researchers said research with larger datasets is needed.